This essay was edited out of a chapter of my book, The Intelligence Illusion: a practical guide to the business risks of Generative AI, with minor alterations.
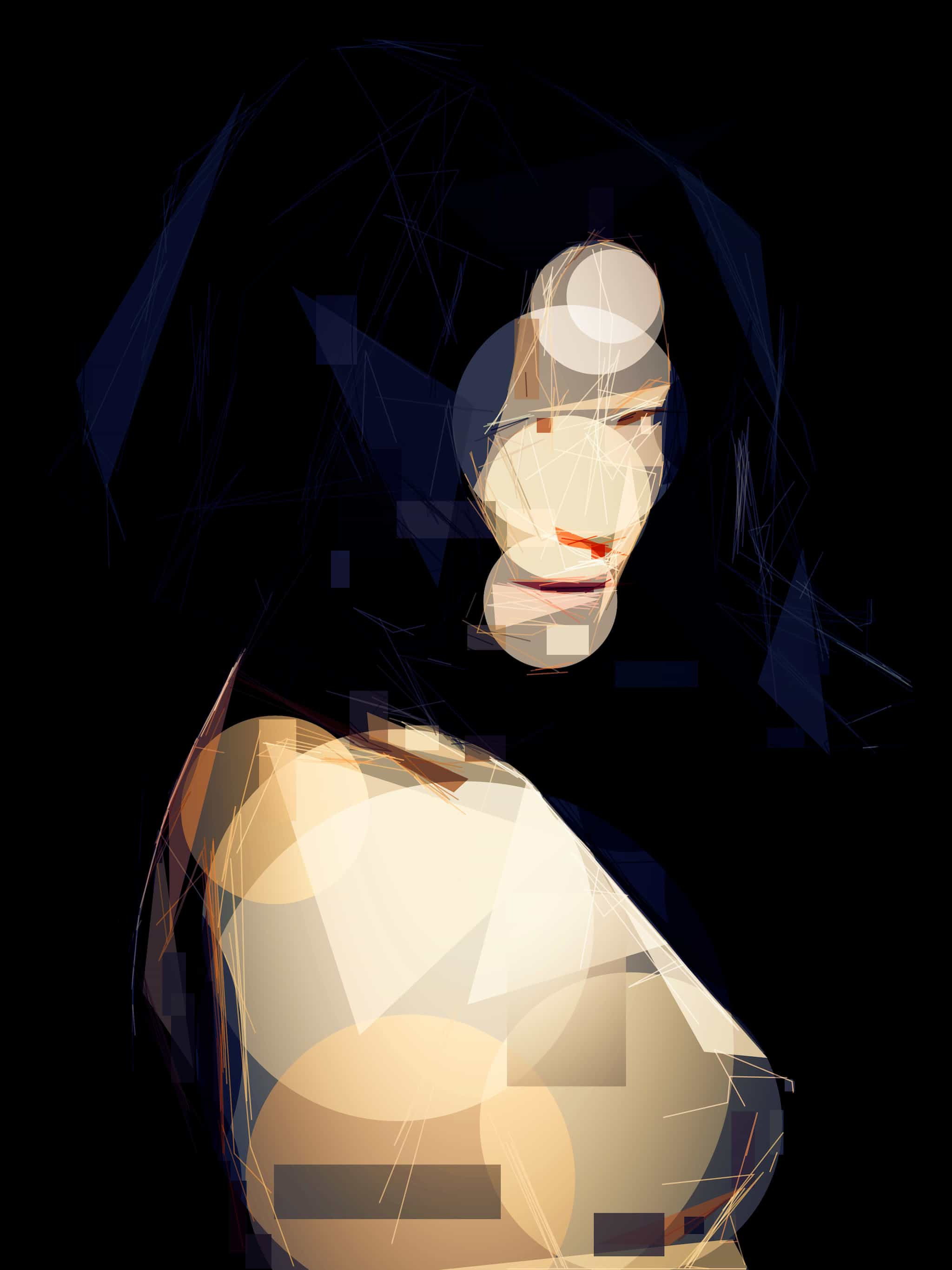
“See the choice of dreams”, and then worry about it
Very well. This book – this side, Dream Machines – is meant to let you see the choice of dreams. Noting that every company and university seems to insist that its system is the wave of the future, I think it is more important than ever to have the alternatives spread out clearly.
But, the experts are not going to be much help, they are part of the problem. On both sides, the academic and the industrial, they are being painfully pontifical and bombastic in the jarring new jargons (see “Babes in Toyland,” p. 4). Little clarity is spread by this. Few things are funnier than the pretensions of those who profess to dignity, sobriety and professionalism of their expert predictions – especially when they too are pouring out their personal views under the guise of technicality. Most people don’t dream of what’s going to hit the fan. And the computer and electronics people are like generals preparing for the last war.
Frankly, I think it’s an outrage making it look as if there’s any kind of scientific basis to these things: there is an underlevel of technicality but like the foundation of a cathedral, it serves only to support what rises from it. THE TECHNICALITIES MATTER A LOT. BUT THE UNIFYING VISION MATTERS MORE.
Ted Nelson, Computer Lib/Dream Machines[1]
AI software development – the “what is this for?” part – has never had much of a unifying vision. AI research, sure, they have a vision: they want intelligent machines first, figure out what to do with them second.
They dream of a robot future.
Some parts of the research monomania end up having clear software benefits. Being able to point a computer at an image and have it get at least a rough idea of what the picture is of is neat and that came from Machine Learning research. It didn’t come with a single specific “what is this for?” vision except, you know, “how is our robot going to see?”, but it made up for it by being obviously useful in a general sort of way. It’s a capability that’s now built into pretty much all of our devices. As a feature that’s now integrated into our lives, it’s a microcosm of the issues we have with innovations coming out of AI research:
- It has helped the blind and partially-sighted access places and media they could not before. A genuine technological miracle.
- It lets our photo apps automatically find all the pictures of Grandpa using facial recognition.
- It has become one of the basic building blocks of an authoritarian police state, given multinational corporations the surveillance power that previously only existed in dystopian nightmares, and extended pervasive digital surveillance into our physical lives, making all of our lives less free and less safe.
One of these benefits is not like the other.
Universal facial recognition is terrifying when it works perfectly and a nightmare when it’s flawed. It exaggerates power imbalances and disproportionally enables bad actors and authoritarians. It’s equal parts pleasant domestic miracles and blighted social and political horror. Generative AI is likely to follow the same path.
In the absence of a unifying vision, the tech industry simply does what makes the most money for people working in the tech industry – greed fills the void where there should have been vision. Companies such as Amazon didn’t hesitate to sell facial recognition services to law enforcement – until the backlash forced them to stop.[2]
This might just be capitalism, but the ‘just’ in that phrase feels quite different when the industry in question is peddling synthetic miracles.
Greed might be inevitable. It might always seep into the cracks, break apart the concrete foundations our ivory towers are built on. But, having a coherent unifying vision that’s backed by clear values does a remarkable job of holding off the decay.
Even today, the web is like living fossil, a preserved relic from a different era. Anybody can put up a website. Anybody can run a business over it. I can build an app or service, send the URL to anybody I like, and most people in the world will be able to run it without asking anybody’s permission. There are rules you have to follow, obviously, but those are remarkably straightforward if you aren’t actively spying on people or messing around with their data – especially when you’re working on a comparatively small scale.
You can trace the lineage of the vision behind the web from Tim Berners-Lee[3] in 1989, through Ted Nelson in 1974 and Douglas Engelbart in 1968, all the way to Vannevar Bush’s article As We May Think in the Atlantic Monthly back in 1945.[4]
All of their books, software prototypes, theories, and ideas run along the single continuing thread of the hypertext concept – links – as a new kind of punctuation mark[5] that connects the information of the world together in a coherent network. It’s a vision that encompasses concept, functionality, interface, and values – and it persists to this day, despite decades of greed, abuse, surveillance, and shitty ads. It’s a unifying vision of a world that’s simultaneously technological and literate. This vision is part of what has kept it alive. Despite the frustrations, pain, and the flaws, working on making parts of the web is a privilege.
While AI researchers are busy trying to build their robot dream, generative AI software has no such unifying vision. Some vendors are bent on replacing humans at their jobs – effectively promoting their software as “AI” illustrators, voice actors,[6] even “robot” lawyers.[7] – and then look surprised at sheer enormity of the anger that they get in response. Other vendors are resurrecting Microsoft’s 1990s dream of intelligent agents, assistants, or copilots who operate in the context of the software you use – extending Clippy’s lineage into the modern world.[8] The rest seem to have lazily reached for the first interface metaphor they could think of – the chatbot – with no thought or even the vaguest idea of how it should actually integrate with the rest of the work we’re doing.
As much as it makes your average MBA salivate, “let’s replace people with something shittier but cheaper” isn’t much of a vision for software development and user interface design, which leaves those of us who are genuinely curious about the applications of the technology with the other two paths.
Those seem to be converging towards a single idea: “Human-in-the-loop”. It’s the idea that in the interactive loop with the AI software, the decision-making, choices, and actions are made by the human.[9] Instead of full automation there’s feedback from the AI as the tasks progress and the human responds to that by interacting with the various user interface affordances provided by the system.
In other words, the human sits in front of software, uses it as they would any other software, and then it does stuff for them as any other software does, except in an AI way.
The grand unifying vision of AI-assisted software is that you should use it to make software.
That’s an idea that’s only remarkable because of how many AI enthusiasts think they can do away with the people part of getting things done.
Acquiesce, or mitigating the inevitable
In an earlier chapter I wrote about the failure of an AI model designed to predict the onset of sepsis, how external reviewers discovered the flaws, which then led to the vendor updating and improving it.
At one hospital, UC Health in Colorado, they found that the system still wasn’t that useful: “the ratio of false alarms to true positives was about 30 to 1”.[10]
To salvage their investment UC Health changed their approach with the system. Instead of using the AI as an autonomous prediction system that sent out alerts to overworked doctors and nurses, they put together a special monitoring team of clinicians that used live video feeds to helped filter out the false alarms. That team built relationships with bedside nurses throughout the hospital. Where the AI system alone was utterly useless, the human team, assisted by their relationships with the nurses and the AI system, was estimated to save about 211 lives annually.
AI on its own was worse than nothing while AI as an assistant saved lives, a clear demonstration of the value of human-in-the-loop. It’s a heart-warming parable that lends credence to the ambitions of those who are trying to make “AI assistants” happen.
This, and other stories like it, are going to be the foundation myths of a thousand new AI services – the seeds of a new computing revolution.
Or, more specifically, it’s a certain kind of fertiliser. The kind that smells.
Case studies are amazing tools. You can pick one instance where everything worked out – an exercise absent of disaster – has a nice “all is lost” moment that gets turned around, and throw together a just-so story that proves exactly the point you want. There isn’t anything anybody can do to disprove it without particle-colliding themselves into an alternate reality. There is no way to ‘science’ a case study unless you have access to a parallel universe as a control. They’re all just stories that short-circuit our thinking.
For every UC Health sepsis story there are a hundred systems that didn’t work. Even the UC Health story itself is dubious once you dig into it. Was it the AI that saved 211 lives? Or was it having a specialised team of clinicians watching all the at-risk patients around the clock, using live feeds? Or, was it the relationships the clinicians developed with the bedside nurses? If you’d put together that same team with the same infrastructure, but using a simpler, cheaper algorithm based on vital sign monitors, would that have done the same job? Why didn’t UC Health try that first – simpler, cheaper, faster to set up – if what they wanted was to save lives?
The answer is simple: they’d already bought a broken AI system. They did what they had to in terms of making sure their investment did eventually save lives, but it leaves us with this unanswered question: if they had spent that same amount of money on building teams and a system for detecting sepsis, but without AI, would it have worked better or worse?
We can’t know, and that’s why case studies are a favoured tool by MBAs, startups, and consultants all over the world. You can just pick a story that proves what you want and ignore the other hundred that don’t.
Once Generative AI becomes a broad movement in software, facts and science won’t matter, and the stories will take over. Ted Nelson was writing about computers and programming in a more general way and in a different era, but he’s right here too: the stories about AI software aren’t scientific and trying to make them look scientific is an outrage.
That’s what the AI software vendors are doing with their marketing performances that look like scientific papers, the ‘studies’ that are little more than sales exercises, and the entirety of their rhetoric about being on the verge of AGI – how we need to make sure those future robot gods are our slaves and not overlords.
It’s storytelling.
Fighting that with another ‘science’ performance is futile. In a war of theatrics, the act with the biggest budget wins the crowd. We can chip away at the foundations with peer-reviewed papers and research that show flaws and failures, but ultimately what will decide this in the decades to come is the software – how well it’s designed, how effective, how productive, and the long-term failures and successes in real workplaces.
That’s where the three core flaws of the assistant model is going to be a problem.
I mentioned two of them earlier, automation and anchoring biases. We, as human beings, have a strong tendency to trust machines over our own judgement.[11] This kills people, as it’s been a major problem in aviation.[12] Anchoring bias comes from our tendency to let the initial perceptions, thoughts, and ideas set the context for everything that follows. AI adds a third issue: anthropomorphism.[13] Even the smartest people you know will fall for this effect as large language models are incredibly convincing.[14] These biases combined lead people to feel even more confident in the AI’s work and believe that it’s done a better job than it has.[15]
We’re using the AI tools for cognitive assistance. This means that we are specifically using them to think less.[16] In every other industry this dynamic inevitably triggers our automation bias and compromises our judgement of the work done by the tools.[17] We use the assistant to think less, so we do.
These models are incredibly fluent and – as we saw at the start of this book – are consistently presented by their vendors as near-AGI. This triggers our instinct towards anthropomorphism,[18] making us feel like we have a fully human-level intelligence assisting us, creating an intelligence illusion that again hinders are ability to properly assess the work it’s doing for us.[19]
Anthropomorphism, when applied to AI chatbots has been called the “Eliza effect”. It was first observed by Joseph Weizenbaum when he saw how people responded to and interacted with the comparatively primitive ‘AI’ chatbot, Eliza, that he created back in 1966.
What I had not realized is that extremely short exposures to a relatively simple computer program could induce powerful delusional thinking in quite normal people.
Joseph Weizenbaum[20], p. 7.
Fluent AI models create an anthropomorphism effect that sways even those who knew that the AI was nothing more than a simplistic program, even by 1966 standards.[21]
The intelligence illusion, the conviction that these are artificial minds capable of powerful reasoning, when combined with anthropomorphism supercharges our automation bias. Our first response to even the most inane pablum from a language model chatbot is awe and wonder. It sounds like a real person at your beck and call! The drive to treat it as, not just a person, but an expert is irresistible. For most people, the incoherence, mediocrity, hallucinations, plagiarism, and biases won’t register over their sense of wonder.
This anthropomorphism-induced delusion is the fatal flaw of all AI assistant and copilot systems. It all but guarantees that – even though the outcome you get from using them is likely to be worse than if you’d done it yourself, because of the flaws inherent in these models – you will feel more confident in it, not less.
Every human-in-the-loop and assistant-style AI system I’ve seen has these defects. Some of them even do their best to exacerbate them by making the assistant adopt a confident tone or an affable demeanour.
Those who use these AI systems are likely to get worse results and still be more confident in the resulting output than they would have in their own. Their work will suffer, but they will feel like it has improved. This is a recipe for fanatical evangelism and incredible revenue growth. It all but guarantees that we’ll see a financial bubble of some kind around AI. The only question is its size and duration. The more effective the Generative AI systems are, the bigger the bubble. The less effective they are, the faster it’ll pop.
We’ll probably get some good software out of it – especially when it comes to converting or modifying text and media – but it’s the nature of bubbles to create crap. A software bubble is the flowering of a thousand first-movers – countless startups and tech companies, most of them utterly clueless about what they’re working with, building the first bad iterations of what they hope is a good idea. We don’t know yet what the ideal, productive AI-assisted productivity software will look like, but we do know that we’re unlikely to see many examples of it in the first generation.
Meanwhile, the tech industry will dream of exponential growth.
The roads home
I have lived abroad for most of the past twenty years. The web let me work wherever I wanted without losing touch with my friends and family. The tools the web offers gave me freedom that I couldn’t have imagined when I was a child.
This worked well for a while. I’ve had the joy of living in a number of wonderful cities and amazing neighbourhoods and communities.
I grew older and, with age, those around you also grow older. Some of them get sicker. A video chat doesn’t fill the void you feel when somebody you care about is lying in a hospital bed. But the freedom the web provides works in the other direction as well. I could live near those I care about and the web meant I could keep doing my job no matter where I was.
I decided to move back home to Iceland. As I was preparing my move, the COVID-19 pandemic struck, and the rest of the world discovered what I had known for decades: the web abstracts distance. You can work where you want. I made my way home, despite the collapse of international airline travel. From Montréal to Toronto. Toronto to Amsterdam. Finally, I flew from Amsterdam to Iceland.
Back in Iceland, I settled in Hveragerði, a small town of about 2700 people in the south of Iceland. Keeping with theme of my realisation that the web and related technologies meant that location mattered less, I could pick a place that suited my personal needs. It’s a nice town. The weather here can be interesting – this is Iceland after all – which often leads to road closures in the winter. But there are three separate roads that connect this region with the capital, so even though a couple of the roads get closed due to snow or ice there’s always the third. Because we know what to expect from the weather, most regions in Iceland invest in their infrastructure. We try to make sure we can keep everything going even when a bad storm hits us. There are redundancies and, for the most part, they work.
We can’t say the same about the software that we have today – that we use for our work. Even though many organisations have returned to the office, partially or fully, we are still using the same software that companies adopted for remote work. We use Google Docs, Zoom, Dropbox, or an equivalent competitor. Our files, documents, and processes are now tied to whatever app we’ve adopted.
If Google Docs goes down or has sporadic outages, then our work disappears with it. If our internet goes, the software blinks out. When the biggest data centre belonging to Amazon Web Services goes down, that breaks most of their services across all of their data centres, because they’re all interconnected, and almost all of our software breaks with it.[22] Given our increasing reliance on centrally hosted software services, the impact of temporarily losing a data centre is severe, getting worse, and is now even happening because of the weather, caused by an increase in frequency and magnitude of heatwaves globally.[23]
It doesn’t matter whether we ourselves are working remotely or in-office, all our software today is remote, and the connection to it can break in a thousand different ways.
There is only one road into town.
A global network means our software shouldn’t have to be centrally located in only a few specific buildings across the world. It should be spread throughout the network, on every device that’s connected to it. Our hardware devices shouldn’t have to be so reliant on the internet that core features cease to function just because they can’t phone home for a short while. Our information – public, private, professional – shouldn’t have to be controlled, collected, and stored by only a handful of corporations.
The software we have today is undermining the strongest advantage given to us by the internet: robust and distributed reliability. Our work depends on increasingly unreliable software. Their need to be always online means you feel every hiccup in your connection. Centralisation means that when something does go wrong, it’s potentially catastrophic as it affects everybody, everywhere, who is using that centralised app. This matters because things are going wrong, fast. There’s political unrest. Social instability. Cold wars. Hot wars. Trade wars. A climate crisis. Data that was just normal personal data one moment, becomes incriminating evidence a moment later when people’s rights are stripped away.
The software we have isn’t the software we need.
The opposite of good software
Modern software is remarkably fragile. We’ve gone from a software ecosystem that, a few years ago, was almost completely local, to one where everything is just cached – temporarily stored – at best. A decade ago what you worked with was on the computer itself. Your data was your own, and relatively safe if you kept decent care of your backup drive. The apps were yours, usually bought and paid for once – no subscription. Collaboration was always a bit tricky if you weren’t a software developer – we’ve always had somewhat decent collaborative tools in version control systems – but other people made do by using shared local servers or simply sending files over email.
This was a remarkably robust software ecosystem that tolerated all sorts of disasters, disconnections, and changes. We’ve dismantled it in less than a decade. Most of the apps we use for our work require an internet connection. Almost all of them are entirely cloud-based, where significant parts of the software runs on a server somewhere. Little of our work data is stored locally any more.
Generative AI serves to accelerate that trend.[24] You needed 800 GB just to store GPT-3, without even running it.[25] Later versions and ChatGPT are even bigger, running in parallel on multiple servers. The technology can be made to work locally, but that’s not where the hype is. The hype is for the already countless “AI for X” services who are all in the cloud and are all using services from OpenAI.[26] Unless one of the big tech companies breaks ranks and builds into their Operating system a solid and tested large language model that has been ethically trained on documented data sets, what we’re going to get are agents and chatbots everywhere, each living in the cloud, fine-tuned for a task, and hooked up to whatever APIs the startups think are nifty. Why solve the hard problem of making a language model safer, better integrated into your software, and more sustainably developed, when you can hook up a finicky but flashy website with OpenAI and call it a startup?
The dream the tech industry chose is not science or progress. The dream they chose is that of easy money, because that’s the only dream the tech industry today is capable of seeing. Their vision is a mirage of craving.
Their want can only be met with another financial bubble, one that has to be more grand and world-changing than any other that preceded it. They crave the exponential to fulfil their dreams, but the only true exponential today’s twenty-something startup founder will experience is that of the escalating Climate Crisis. That won’t stop them from trying. Their hunger is likely to push them to ignore the social unrest and power shifts that AI systems cause.
The tech industry doesn’t just behave with your normal corporate greed. They want financial bubbles. They had a taste of the euphoria with the dot-com bubble and the hunger for it never went away.
The tech industry is also, as I argued at the start of this book, full of true believers in AI. Somebody who truly believes – sincerely believes that this will all be for the best – will push past the mass unemployment, organised disinformation, and wholesale deception. They will think that it will all be worth it. Once we get through the initial “disruption”, things will be better for everybody.
None of this is conducive to software design and development. It isn’t a mindset that leads you to do user research, observational studies, or usability experiments. It’s a drive that’s taking them away from what most people and their communities need. Where we need robust technology, they are giving us finicky AIs that misbehave at a badly worded sentence. Where we need privacy from both corporations and potentially hostile authorities, they push further and further into recording our lives. When we need software that works on the devices we have, for as long as they last, they give us software that only works on the latest and greatest. Sometimes, as with GPT-4, the software they make even requires systems so powerful that they only exist in a couple of locations on the planet.
But, don’t worry, they’ll sell us access – timeshare, really – but let’s call it “the cloud”. It only breaks some of the time.
Nothing they do is for us, even though it’s our money, our data, and our art, writing, and music they’re demanding. We aren’t customers to them – we’re just the people that pay. To tech companies, we are nothing more than a resource to be tapped. A number to be boosted to pump investor interest. They are not doing us any favours. What they want from us is simple: everything. All culture on their servers, made by their AI. All our work happening through them, assisted by their AI. The totality of our information, mediated by their AI. A vig collected on all existence.
One of the papers I’ve referred to a few times in this book is On the Dangers of Stochastic Parrots: Can Language Models Be Too Big?[27] It was the first paper to provide a cohesive and detailed overview of how large language models work, how they affect people, and the risks that they pose. This paper ultimately led to Google firing one of the key authors of the paper, Timnit Gebru, and forced other co-authors employed by Google to take their name off it.[28] This continues to this day, where Google employees seem to be routinely discouraged from working on AI fairness or ethics.[29]
When Microsoft launched Bing Chat, the first mainstream attempt to use a large language model as a front end for search – something that another co-author of On Stochastic Parrots, Emily M. Bender, had warned against in a separate paper titled Situating Search[30] – this lead to the exact outcomes they had predicted. Strange behaviour[31], threatening language[32], falsehoods[33] and lies[34] ensued. Bing Chat played out exactly the way they expected.
Of course, Microsoft did the only rational thing it could when the risks of its products were revealed: it disbanded its AI ethics and safety team[35] and rolled Bing Chat out to even more people.[36] It now plans to push towards adding AI chatbots to everything, everywhere, no matter the cost.[37]
Most of the tech organisations that had responsible AI or AI safety teams are disbanding them.[38]
They seem to think it would be a mistake to worry about risks and problems – why worry about something you can probably fix?[39] Who cares about the harm it does in the meantime?
Safe, for the tech industry, is too slow when you hunger for a bubble and want to ship more software, to more people, as fast as you can.
Designers of software user interfaces often imagine deliberately bad designs as an exercise – a way of demonstrating the principles of their craft by exploring their opposites. It’s a good way of demonstrating why a design principle matters, and it can provide tactile examples of who benefits from it and how.
If you asked me to imagine the software that would be the opposite of what we need as a society…
That app would look remarkably like ChatGPT.
The best way to support this newsletter or my blog is to buy one of my books, The Intelligence Illusion: a practical guide to the business risks of Generative AI or Out of the Software Crisis.
Ted Nelson, Computer Lib/Dream Machines (Place of publication not identified, 1974). ↩︎
Jeffrey Dastin and Jeffrey Dastin, “Amazon Extends Moratorium on Police Use of Facial Recognition Software,” Reuters, May 2021, https://www.reuters.com/technology/exclusive-amazon-extends-moratorium-police-use-facial-recognition-software-2021-05-18/. ↩︎
“A Little History of the World Wide Web,” accessed April 6, 2023, https://www.w3.org/History.html. ↩︎
Vannevar Bush, “As We May Think,” The Atlantic, July 1945, https://www.theatlantic.com/magazine/archive/1945/07/as-we-may-think/303881/. ↩︎
Stacey Mason and Mark Bernstein, “On Links: Exercises in Style,” in Proceedings of the 30th ACM Conference on Hypertext and Social Media, HT ’19 (New York, NY, USA: Association for Computing Machinery, 2019), 103–10, https://doi.org/10.1145/3342220.3343665. ↩︎
Joseph Cox, “‘Disrespectful to the Craft:’ Actors Say They’re Being Asked to Sign Away Their Voice to AI,” Vice, February 2023, https://www.vice.com/en/article/5d37za/voice-actors-sign-away-rights-to-artificial-intelligence. ↩︎
“DoNotPay - The World’s First Robot Lawyer,” accessed April 6, 2023, https://donotpay.com/. ↩︎
Benjamin Cassidy, “The Twisted Life of Clippy,” Seattle Met, August 2022, https://www.seattlemet.com/news-and-city-life/2022/08/origin-story-of-clippy-the-microsoft-office-assistant. ↩︎
Ge Wang and Juliana Bidadanure, “Humans in the Loop: The Design of Interactive AI Systems,” Stanford HAI, October 2019, https://hai.stanford.edu/news/humans-loop-design-interactive-ai-systems. ↩︎
Casey Ross, “Epic’s Overhaul of a Flawed Algorithm Shows Why AI Oversight Is a Life-or-Death Issue,” STAT, October 2022, https://www.statnews.com/2022/10/24/epic-overhaul-of-a-flawed-algorithm/. ↩︎
Raja Parasuraman and Victor Riley, “Humans and Automation: Use, Misuse, Disuse, Abuse,” Human Factors: The Journal of the Human Factors and Ergonomics Society 39, no. 2 (June 1997): 230–53, https://doi.org/10.1518/001872097778543886. ↩︎
Kathleen L. Mosier et al., “Automation Bias: Decision Making and Performance in High-Tech Cockpits,” The International Journal of Aviation Psychology 8, no. 1 (January 1998): 47–63, https://doi.org/10.1207/s15327108ijap0801_3. ↩︎
Arvind Narayanan and Sayash Kapoor, “People Keep Anthropomorphizing AI. Here’s Why,” Substack newsletter, AI Snake Oil, February 2023, https://aisnakeoil.substack.com/p/people-keep-anthropomorphizing-ai. ↩︎
Murray Shanahan, “Talking About Large Language Models” (arXiv, February 2023), https://doi.org/10.48550/arXiv.2212.03551. ↩︎
Neil Perry et al., “Do Users Write More Insecure Code with AI Assistants?” (arXiv, December 2022), https://doi.org/10.48550/arXiv.2211.03622. ↩︎
K. Mosier and L. Skitka, “Human Decision Makers and Automated Decision Aids: Made for Each Other?” 1996, https://www.semanticscholar.org/paper/Human-Decision-Makers-and-Automated-Decision-Aids%3A-Mosier-Skitka/ffb65e76ac46fd42d595ed9272296f0cbe8ca7aa. ↩︎
Kathleen L. Mosier et al., “Automation Bias, Accountability, and Verification Behaviors,” Proceedings of the Human Factors and Ergonomics Society Annual Meeting 40, no. 4 (October 1996): 204–8, https://doi.org/10.1177/154193129604000413. ↩︎
See Nicholas Epley, Adam Waytz, and John T. Cacioppo, “On Seeing Human: A Three-Factor Theory of Anthropomorphism,” Psychological Review 114, no. 4 (October 2007): 864–86, https://doi.org/10.1037/0033-295X.114.4.864, which outlines three psychological triggers for anthropomorphism: 1. If you don’t know how a non-human agent works, we default to thinking it works like us because that’s what we have the most familiarity with. 2. “The motivation to interact effectively with nonhuman agents” causes us to attribute human characteristics and motivation. 3. Seeing agents as human-like enables “a perceived humanlike connection with nonhuman agents.” ↩︎
Arleen Salles, Kathinka Evers, and Michele Farisco, “Anthropomorphism in AI,” AJOB Neuroscience 11, no. 2 (April 2020): 88–95, https://doi.org/10.1080/21507740.2020.1740350, esp. “In the general public it inadvertently promotes misleading interpretations of and beliefs about what AI is and what its capacities are.” Anthropomorphism also limits the researchers, which is important to note in light of the common belief in the field that the spark of AGI has been struck: “Furthermore, anthropomorphic (implicit or explicit) interpretations of AI might also have epistemological impact on the AI research community itself, insofar as the search for biological and psychological realism (i.e., similarity with biological intelligence) might lead to underestimating the possibility of new theoretical and operational paradigms and frameworks thus ultimately limiting the development of AI.” ↩︎
Computer Power and Human Reason: From Judgment to Calculation (San Francisco: Freeman, 1976). ↩︎
Weizenbaum, 6. ↩︎
Mike Moore and Joel Khalili last updated, “AWS Went down Hard, yet Again - Here’s What Happened,” TechRadar, December 2021, https://www.techradar.com/news/live/aws-is-down-again-heres-all-we-know. ↩︎
Nicholas Fearn, “Heat Waves Are Shutting Down Data Centers and Breaking the Internet,” Gizmodo, December 2022, https://gizmodo.com/heat-waves-climate-change-data-center-server-shut-down-1849916741. ↩︎
Sarah Myers West, “Competition Authorities Need to Move Fast and Break up AI,” Financial Times, April 2023. “Without the robust enforcement of competition laws, generative AI could irreversibly cement Big Tech’s advantage, giving a handful of companies power over technology that mediates much of our lives.” ↩︎
“GPT-3,” Wikipedia, April 2023, https://en.wikipedia.org/w/index.php?title=GPT-3&oldid=1147823352. ↩︎
James Governor, “The Great Flowering: Why OpenAI Is the New AWS and the New Kingmakers Still Matter.” James Governor’s Monkchips, April 2023, https://redmonk.com/jgovernor/2023/04/13/the-great-flowering-why-openai-is-the-new-aws-and-the-new-kingmakers-still-matter/. ↩︎
Emily M. Bender et al., “On the Dangers of Stochastic Parrots: Can Language Models Be Too Big?” in Proceedings of the 2021 ACM Conference on Fairness, Accountability, and Transparency, FAccT ’21 (New York, NY, USA: Association for Computing Machinery, 2021), 610–23, https://doi.org/10.1145/3442188.3445922. ↩︎
Karen Hao, “We Read the Paper That Forced Timnit Gebru Out of Google. Here’s What It Says.” MIT Technology Review, 2020, https://www.technologyreview.com/2020/12/04/1013294/google-ai-ethics-research-paper-forced-out-timnit-gebru/. ↩︎
Davey Alba and Julia Love, “Google’s Rush to Win in AI Led to Ethical Lapses, Employees Say,” Bloomberg.com, April 2023, https://www.bloomberg.com/news/features/2023-04-19/google-bard-ai-chatbot-raises-ethical-concerns-from-employees, “Even after the public pronouncements, some found it difficult to work on ethical AI at Google. One former employee said they asked to work on fairness in machine learning and they were routinely discouraged — to the point that it affected their performance review. Managers protested that it was getting in the way of their ‘real work,’ the person said.” ↩︎
Chirag Shah and Emily M. Bender, “Situating Search,” in ACM SIGIR Conference on Human Information Interaction and Retrieval, CHIIR ’22 (New York, NY, USA: Association for Computing Machinery, 2022), 221–32, https://doi.org/10.1145/3498366.3505816. ↩︎
Simon Willison, “Thoughts and Impressions of AI-Assisted Search from Bing,” February 2023, http://simonwillison.net/2023/Feb/24/impressions-of-bing/. ↩︎
“Microsoft’s New ChatGPT AI Starts Sending ‘Unhinged’ Messages to People,” The Independent, February 2023, https://www.independent.co.uk/tech/chatgpt-ai-messages-microsoft-bing-b2282491.html; Simon Willison, “Bing: ‘I Will Not Harm You Unless You Harm Me First’,” 2023, http://simonwillison.net/2023/Feb/15/bing/. ↩︎
Dmitri Brereton, “Bing AI Can’t Be Trusted,” February 2023, https://dkb.blog/p/bing-ai-cant-be-trusted. ↩︎
Nick Diakopoulos, “Can We Trust Search Engines with Generative AI? A Closer Look at Bing’s Accuracy for News Queries,” Medium, February 2023, https://medium.com/@ndiakopoulos/can-we-trust-search-engines-with-generative-ai-a-closer-look-at-bings-accuracy-for-news-queries-179467806bcc. ↩︎
Zoë Schiffer, “Microsoft Just Laid Off One of Its Responsible AI Teams,” March 2023, https://www.platformer.news/p/microsoft-just-laid-off-one-of-its. ↩︎
Tom Warren, “You Can Play with Microsoft’s Bing GPT-4 Chatbot Right Now, No Waitlist Necessary,” The Verge, March 2023, https://www.theverge.com/2023/3/15/23641683/microsoft-bing-ai-gpt-4-chatbot-available-no-waitlist. ↩︎
Aaron Holmes and Kevin McLaughlin, “Ghost Writer: Microsoft Looks to Add OpenAI’s Chatbot Technology to Word, Email,” The Information, January 2023, https://www.theinformation.com/articles/ghost-writer-microsoft-looks-to-add-openais-chatbot-technology-to-word-email; Benj Edwards, “Microsoft Aims to Reduce ‘Tedious’ Business Tasks with New AI Tools,” Ars Technica, March 2023, https://arstechnica.com/information-technology/2023/03/microsoft-brings-chatgpt-style-ai-to-developer-and-analysis-tools/. ↩︎
Gerrit De Vynck and Will Oremus, “As AI Booms, Tech Firms Are Laying Off Their Ethicists,” Washington Post, March 2023, https://www.washingtonpost.com/technology/2023/03/30/tech-companies-cut-ai-ethics/; Will Knight, “Elon Musk Has Fired Twitter’s ‘Ethical AI’ Team,” Wired, accessed April 27, 2023, https://www.wired.com/story/twitter-ethical-ai-team/. ↩︎
Nico Grant and Karen Weise, “In A.I. Race, Microsoft and Google Choose Speed Over Caution,” The New York Times, April 2023, https://www.nytimes.com/2023/04/07/technology/ai-chatbots-google-microsoft.html. ↩︎